Understanding Agentic AI: The Future of Autonomous Decision-Making
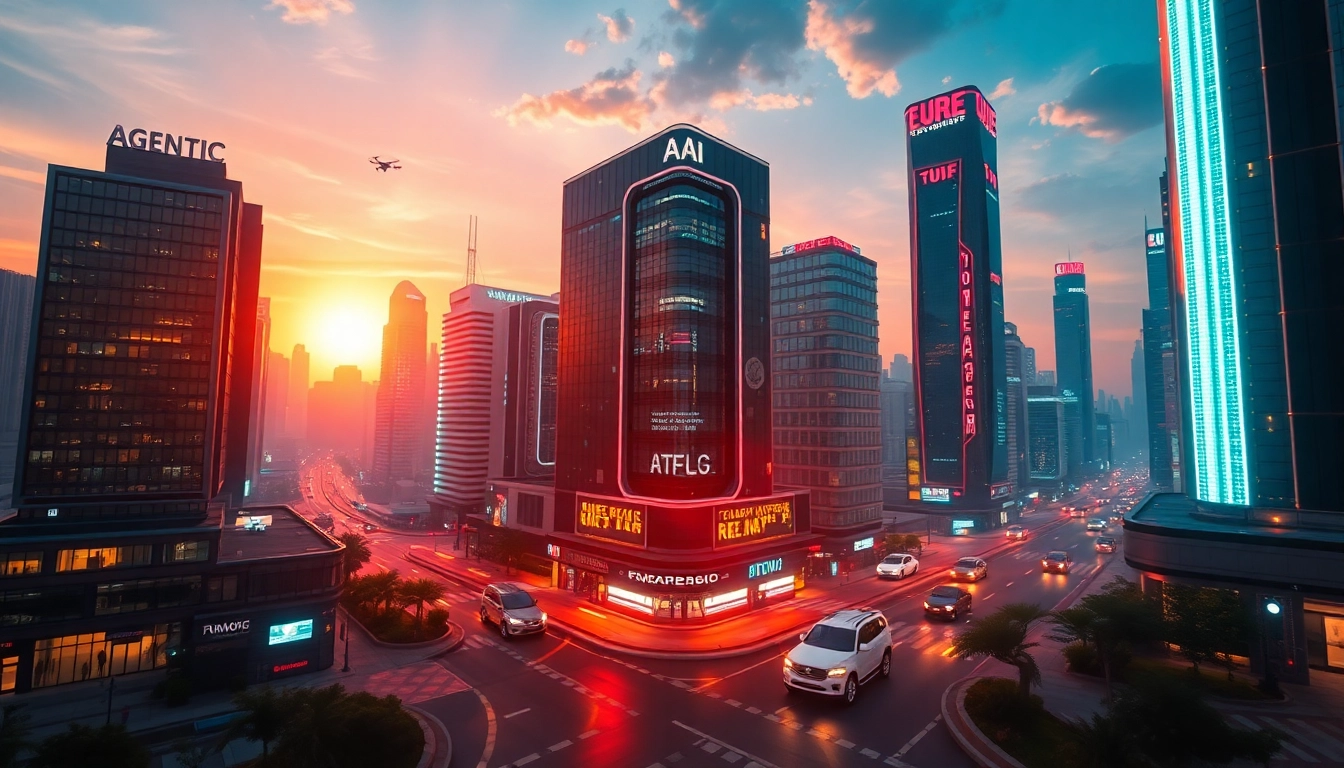
Introduction to Agentic AI
In today’s rapidly evolving technological landscape, Agentic AI stands out as a pioneering concept that promises to transform various sectors by enabling autonomous decision-making capabilities. This artificial intelligence model is characterized by its ability to act independently, plan complex tasks over multiple steps, and adapt to dynamic environments without requiring constant human oversight. As the digital age advances, understanding the implications and applications of Agentic AI can provide organizations with a significant competitive edge.
What is Agentic AI?
Agentic AI refers to artificial intelligence systems endowed with autonomy, capable of making decisions, executing tasks, and learning from their experiences. This type of AI is engineered to handle complex objectives that necessitate a sophisticated understanding of contextual variables and the capacity to operate without direct human intervention. Unlike traditional AI models that rely primarily on set algorithms and require human input for operation, Agentic AI excels in multi-step reasoning, self-initiated planning, and ongoing adaptation.
The Importance of Autonomy in AI
The significance of autonomy in AI lies in its potential to streamline processes, enhance efficiency, and drive innovation. Autonomous systems can operate continuously, providing solutions to complex problems faster than human counterparts. Moreover, in environments where rapid decision-making is critical—like healthcare, finance, or logistics—Agentic AI can outperform traditional models by minimizing human error and maximizing operational speed.
Overview of Key Technologies
Several technologies underpin the effectiveness of Agentic AI, including machine learning, natural language processing, and robotics. Machine learning allows these systems to analyze vast datasets, identify patterns, and make predictions. Natural language processing enables communication in human language, facilitating interactions between humans and AI. Robotics combines these technologies to offer physical embodiments of Agentic AI capable of performing tasks in the real world, from warehouse management to autonomous driving.
The Mechanisms Behind Agentic AI
Decision-Making Processes
At the core of Agentic AI is its decision-making mechanism, which integrates various methodologies to arrive at sound conclusions. These processes can be broken down into several stages:
- Data Input: Agentic AI systems receive inputs from diverse sources, including sensors, past data, and real-time user interactions.
- Analysis: Employing advanced analytics and machine learning algorithms, these systems evaluate the data to identify relevant patterns and trends.
- Planning: Based on the analysis, the AI formulates multiple action strategies to achieve specific goals.
- Execution: The chosen strategy is executed, often with real-time adjustments based on ongoing feedback.
- Learning: After execution, the system analyzes its performance, retaining knowledge for future decision-making improvements.
Iterative Planning and Adaptation
Agentic AI employs iterative planning, allowing for repetitive cycles of decision-making and execution that enhance adaptability. This approach is particularly beneficial in unpredictable environments where conditions change frequently. For instance, in logistics management, an AI system might continually reassess shipping routes based on traffic conditions, weather forecasts, and other external factors, making real-time adjustments to optimize delivery times.
Case Studies of Successful Implementations
To demonstrate the capabilities of Agentic AI, several case studies illustrate its successful deployment:
- Autonomous Vehicles: Companies like Waymo and Tesla utilize Agentic AI to enable cars to navigate complex urban environments, make real-time decisions, and adapt to unforeseen obstacles.
- Healthcare Diagnostics: IBM’s Watson analyzes medical data to assist doctors in diagnosing patients while continuously learning from new cases, improving its accuracy over time.
- Supply Chain Optimization: Businesses like Amazon leverage Agentic AI to manage and monitor supply chain logistics, responding to shifts in demand and optimizing inventory levels dynamically.
Comparing Agentic AI with Other AI Models
Agentic AI vs Generative AI
While both Agentic AI and generative AI leverage advanced algorithms, they serve distinct purposes. Generative AI focuses on creating new content—such as text, images, or music—based on input data. In contrast, Agentic AI is centered on taking actions to achieve specific goals. For example, a generative AI model might write a poem or generate a piece of art, whereas an Agentic AI model might use its reasoning capabilities to manage production in a factory or optimize resource allocation in a business.
Limitations of Traditional AI Systems
Traditional AI systems exhibit several limitations, including:
- Dependence on Large Datasets: Many traditional models require extensive datasets for training, which can be a significant barrier to entry for smaller organizations.
- Lack of Real-Time Adaptability: Traditional systems often struggle to adapt to real-time data changes, leading to outdated responses or predictions.
- Limited Scope of Decision-Making: These systems typically operate within predefined parameters, lacking the flexibility to make autonomous decisions beyond their programmed capabilities.
The Unique Edge of Agentic AI
Agentic AI addresses these limitations head-on. By utilizing advanced algorithms and learning capabilities, it empowers organizations to navigate complexities with minimal human intervention, providing a decisive advantage in rapidly changing environments. For instance, its self-learning nature enables it to glean ongoing insights from trends not previously considered, making it a valuable tool for both strategic and tactical decision-making.
Applications of Agentic AI in Various Industries
Healthcare Innovations
The healthcare sector is witnessing a revolution due to Agentic AI. Its applications range from predictive analytics for patient outcomes to robotic-assisted surgeries. By analyzing historical patient data, these systems can predict the likelihood of diseases, facilitating early interventions. Furthermore, automated diagnostic tools can assist doctors in identifying diseases more accurately and quickly than traditional methods.
Enhancements in Business Operations
Businesses across industries are optimizing their operations with Agentic AI. Customer service chatbots, powered by this technology, can provide seamless and personalized customer experiences, addressing inquiries and resolving issues without human involvement. In the realm of marketing, AI-driven analytics predict customer behavior and improve targeting strategies, resulting in higher conversion rates and customer satisfaction.
Transformations in Smart Cities
Smart city initiatives increasingly rely on Agentic AI to enhance urban living. This technology powers traffic management systems that adapt in real-time to road conditions, public transportation efficiency, and energy consumption management. By harnessing data from various urban infrastructures, Agentic AI can streamline services and improve citizens’ quality of life, creating more sustainable and efficient environments.
The Future of Agentic AI
Trends to Watch in AI Development
The future of Agentic AI appears promising, with several key trends projected to shape its development:
- Increased Integration Across Platforms: We can expect more seamless integration of Agentic AI capabilities across different platforms and industries, enabling significant cross-sector efficiencies.
- Heightened Focus on Privacy and Security: As autonomous systems become more prevalent, developing robust security measures will be paramount to safeguard sensitive data against breaches.
- Regulatory Developments: Governments will likely enact regulations surrounding the use of Agentic AI, particularly in sensitive fields like healthcare and finance, necessitating compliance and ethical considerations.
Ethical Considerations and Challenges
As Agentic AI continues to evolve, ethical considerations are paramount. Concerns regarding bias in data sets, accountability in decision-making, and potential job displacement must be addressed proactively. Efforts to ensure transparency in how these systems operate and make decisions will be crucial in fostering public trust and acceptance.
The Role of Agentic AI in Human Society
Ultimately, the role of Agentic AI within human society will depend significantly on collaboration between technology developers, policymakers, and end-users. Encouraging a conversation about the benefits and risks associated with autonomous systems ensures they are harnessed responsibly and effectively. By integrating human oversight with AI capabilities, organizations can leverage the strengths of both human intelligence and machine autonomy, paving the way for innovation and societal advancement.
Leave a Reply