Maximizing Your Efficiency with AI Search: Techniques for Enhanced Results
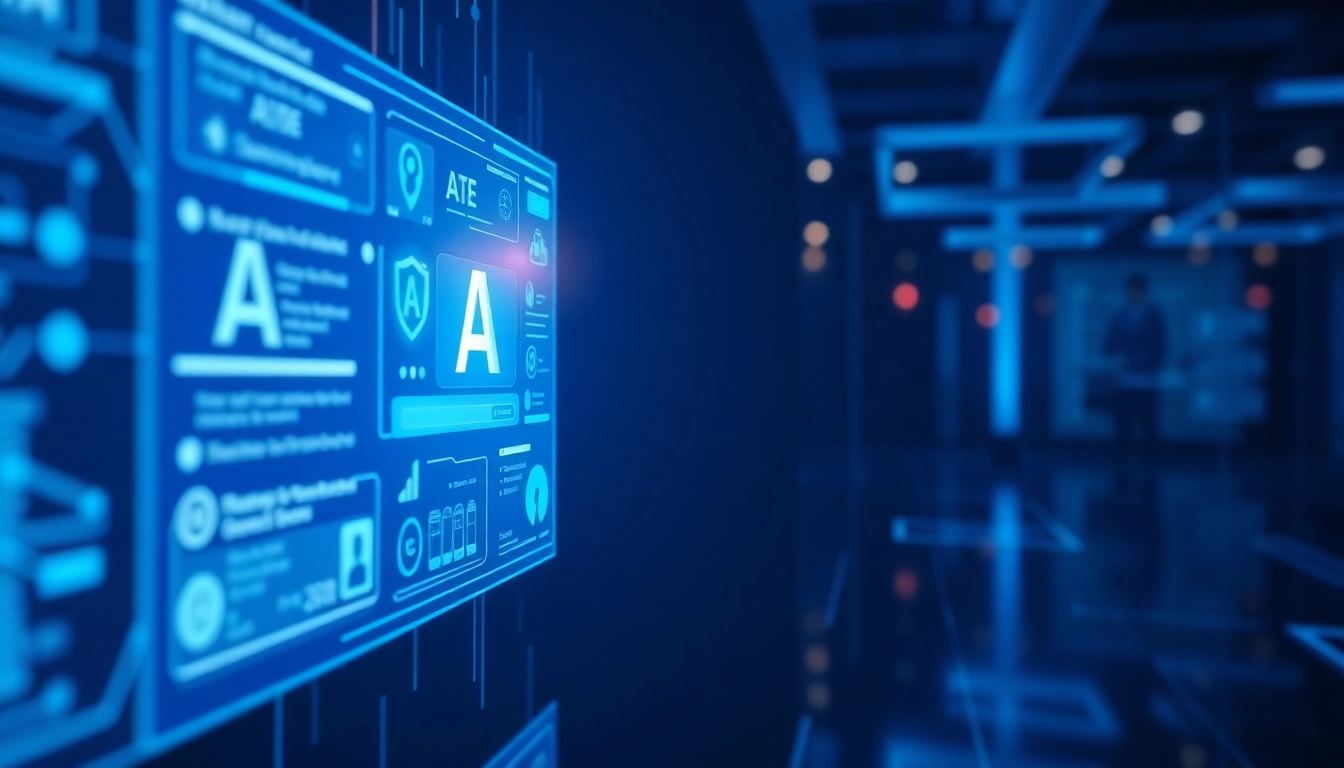
Understanding the AI Search Landscape
The integration of artificial intelligence into search technologies is gradually transforming the way users interact with digital information. AI search engines are not just about retrieving data; they aim to understand context, intent, and the complex nuances of language to provide users with more relevant results. Unlike traditional search engines, which predominantly rely on keyword matching, AI search utilizes sophisticated algorithms to interpret and process queries. This paradigm shift is indicative of a broader trend toward more intelligent and interactive search experiences. By leveraging AI search technologies, businesses and users can enhance their information retrieval processes significantly.
What is AI Search?
AI search refers to the application of artificial intelligence technologies—such as Natural Language Processing (NLP), machine learning, and advanced data analysis techniques—into search engines and information retrieval systems. Unlike traditional search engines that rely on algorithms primarily focused on keyword frequency and linking, AI search aims to comprehend user intentions and the contextual meaning of queries. This capability enables AI-powered search tools to provide answers rather than just a list of links, enhancing user satisfaction and engagement.
The Evolution of Search Engines
The journey of search engines began in the early 1990s with basic keyword-based algorithms. Early search engines could only index and rank web pages based on the occurrences of search terms. This limited understanding often resulted in irrelevant or generic results.
As internet usage grew and information exploded, the need for more sophisticated search mechanisms led to the introduction of PageRank by Google and the growth of semantic search. The past few years have seen a transformative leap toward AI-driven capabilities, building tools that not only index information but also learn from user behavior, trends, and data patterns. This evolution indicates a marketplace that values not just the quantity of information retrieved, but the quality and relevance of that information.
Key Players in the AI Search Market
The landscape of AI search is rapidly evolving, with numerous major players driving innovation:
- Google: As one of the leaders in the AI search space, Google has integrated AI technologies into its search algorithms, enhancing results with tools like Google Lens and the Gemini platform, which serve multimodal capabilities.
- Microsoft: Through offerings such as Bing AI and Azure AI, Microsoft is leveraging AI to provide users with personalized search experiences powered by advanced machine learning.
- OpenAI: With integrations like ChatGPT search, OpenAI is redefining interactivity in search by providing context-aware, conversational answers to user queries.
- Others: Emerging players like Perplexity and iAsk are also making strides by offering innovative search functionalities that emphasize human-like interaction.
Benefits of Integrating AI Search
Improved Accuracy in Results
One of the most significant benefits of AI search is its ability to deliver more accurate and relevant search results. By understanding user intent through advanced algorithms, AI search engines can differentiate between differing meanings of terms and provide results that better align with user needs. For example, a search for “jaguar” could yield entirely different outcomes based on whether the search is related to a car versus the animal.
Machine learning models allow AI search to continuously adapt based on user feedback and behavioral data, enhancing the precision of results over time. This iterative learning process results in fewer irrelevant results and saves users time and effort.
Faster Data Retrieval
The efficiency of AI search engines in processing vast amounts of data allows for quicker retrieval of information. Because AI search uses specialized algorithms to immediately interpret queries and analyze datasets, users can receive answers almost instantaneously. This speed is particularly beneficial in business environments, where timely access to information can inform decision-making.
Moreover, AI search engines optimize background processes, enabling seamless interactions that reduce refresh times, thus providing a smoother overall user experience.
Enhanced User Experience
AI search enhances user experience by offering advanced features such as voice search, personalized results, and predictive suggestions. These capabilities help users navigate through information more intuitively, catering to varied preferences and minimizing frustration.
By implementing conversational interfaces, users can engage naturally with search technologies, asking questions as they would in normal conversations. Such interactions make the search process feel more organic and accessible, aligning well with modern digital behaviors.
Challenges Faced with AI Search Technologies
Data Privacy Concerns
As AI search engines leverage extensive data to refine results, concerns regarding data privacy and security have amplified. User data collection—necessary for personalization—raises questions about consent, ownership, and potential misuse. Organizations must navigate balancing the need for data to drive AI effectiveness against the imperative to protect user privacy. Regulations such as GDPR are critical in shaping how data is handled within AI technologies.
Algorithm Bias and Transparency
AI algorithms are susceptible to biases based on the datasets used in their training. If these datasets contain inherent biases, the algorithms may propagate these biases in search results, leading to skewed information representations. Addressing this challenge requires ongoing scrutiny and intentional measures by developers to ensure fairness, equity, and broad inclusivity in AI models.
Citation Accuracy Issues
One recurring challenge in AI search involves citation accuracy. Many AI search engines struggle to provide reliable sources or context associated with their answers, leading to misinformation. Scholars and professionals often require verifiable information, making an accurate citation essential. Correcting this shortcoming involves developing systems that not only retrieve information but also assess its credibility comprehensively.
Key Technologies Driving AI Search Innovations
Natural Language Processing (NLP)
Natural Language Processing is the driving force behind the conversation capabilities of AI search. By interpreting human language’s complexities—such as slang, idioms, and context—NLP allows search engines to facilitate more human-like interactions. Recent advancements in NLP, such as transformer models, have revolutionized AI, enabling machines to understand and generate human language with remarkable accuracy.
Machine Learning Fundamentals
Machine learning underpins the adaptive nature of AI search algorithms. By using large datasets to identify patterns and make predictions, these algorithms improve their effectiveness through experience. The more interactions users have with an AI search tool, the better the model becomes at anticipating user needs and preferences, creating a refined and personalized search experience.
Retrieval-Augmented Generation (RAG)
Retrieval-Augmented Generation represents a new frontier in AI search technologies by combining traditional retrieval methods with generative capabilities. This method allows the search engine to pull relevant information from multiple sources while also generating explanations or summaries. Consequently, users receive not just a list but coherent answers that synthesize information from various datasets, enhancing both the depth and breadth of responses.
Future Trends in AI Search
Emerging Technologies to Watch
As the landscape of AI search continues evolving, several emerging technologies hold promising potential. Innovations like multimodal search engines, which process various data types (text, images, audio) simultaneously, are already in development. Quantum computing, which can analyze vast datasets at unprecedented speeds, may further enhance AI search capabilities, offering real-time insights even as data complexity grows.
Potential Use Cases Across Industries
AI search technology is applicable across numerous industries. In healthcare, AI search engines can assist in diagnosing diseases by aggregating patient data and suggesting treatments based on available research. In finance, they can streamline compliance checks by rapidly sifting through regulations and legal documents, identifying relevant sections, and assessing potential risks.
Transformations in User Behavior
As AI search technologies evolve, user expectations and behaviors will also transform. Users will increasingly prefer immediate, interactive results over traditional search results. This shift might result in a further decline in traditional keyword searches as users adapt to more conversational search interfaces. To meet these evolving expectations, businesses and developers must anticipate the changing dynamics of user interaction with information retrieval systems.
Leave a Reply