Protect Yourself: Effective Strategies Against Instagram Hacker Threats
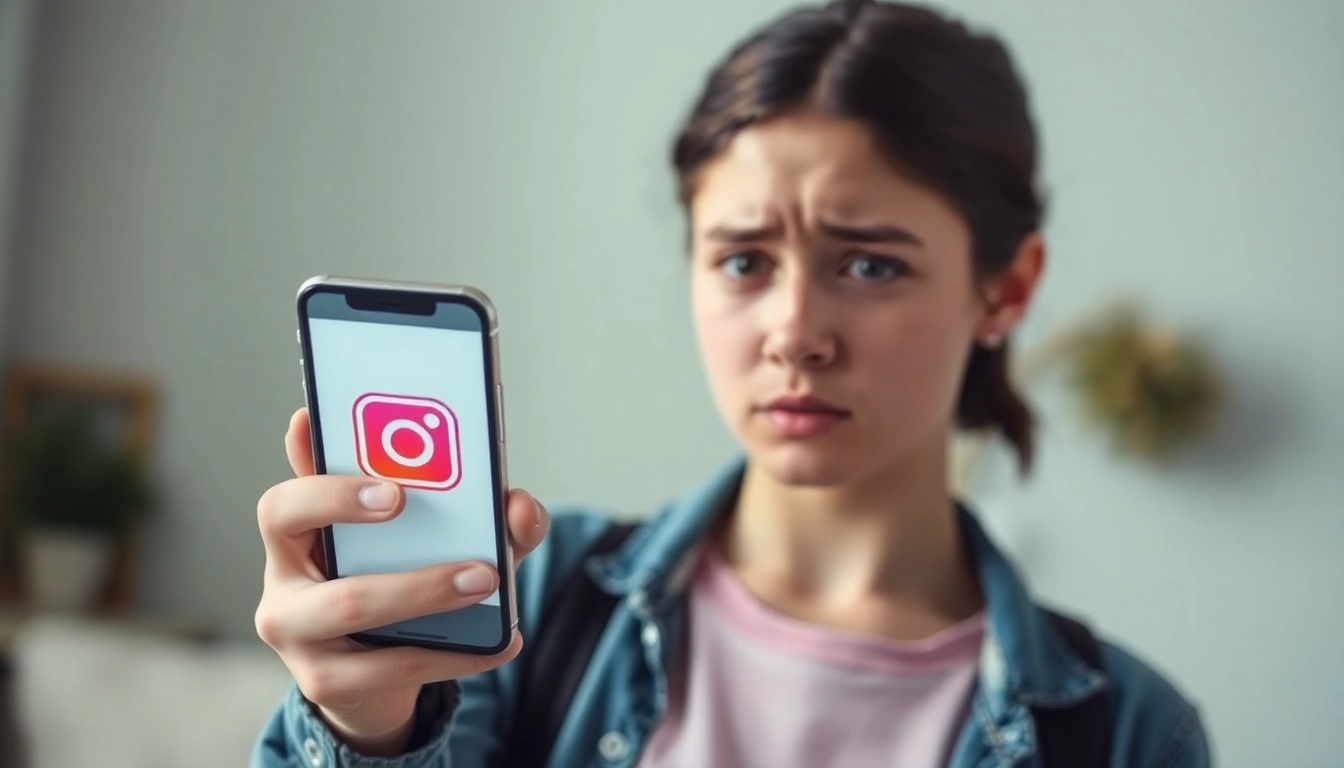
Understanding Instagram Hacking
What is Instagram Hacking?
Instagram hacking refers to unauthorized access to someone’s Instagram account, allowing hackers to manipulate the account for various malicious purposes. This can range from stealing personal information to posting unwanted content or phishing other users. Many individuals and businesses find themselves vulnerable to Instagram hackers due to inadequate security practices. The rise of social engineering tactics and malware has made hacking Instagram accounts alarmingly easy for cybercriminals. Understanding what Instagram hacking entails is crucial for protecting one’s online presence.
Common Methods Used by Instagram Hackers
Hackers exploit a variety of methods to compromise Instagram accounts. Some of the most common tactics include:
- Phishing Attacks: Hackers often use deceptive emails or messages that appear to be from Instagram, prompting users to enter their login information on fraudulent websites. This is a prevalent method due to its effectiveness in luring unsuspecting individuals.
- Brute Force Attacks: In this scenario, hackers use software tools to guess passwords. They may rely on personal information or widely used passwords, making weak passwords a significant vulnerability.
- Social Engineering: This involves manipulating individuals into divulging confidential information. Hackers might pose as Instagram support or even friends to gain trust and access sensitive data.
- Malware: If a user unknowingly installs malicious software on their device, hackers can gain access to their Instagram credentials and other sensitive information.
The Impact of Hacking on Users
The consequences of having an Instagram account hacked can be far-reaching. Users may suffer from:
- Loss of Control: Once a hacker gains access, they can alter account settings, post inappropriate content, or even lock the owner out of their account entirely.
- Data Theft: Hackers can steal personal information, which may lead to identity theft or financial loss.
- Reputational Damage: If a hacked account is used to post negative content or spam, it can harm the individual’s or business’s reputation.
- Emotional Distress: The violation of privacy and potential loss of personal content can lead to significant emotional distress for users.
Signs Your Instagram Account is Hacked
Notable Account Behavior Changes
Recognizing the signs of hacking is the first step toward recovery. Users should be alert for any unusual behavior on their accounts, such as:
- Unfamiliar posts being made without the owner’s consent.
- Messages sent to followers that the owner did not initiate.
- Changes in password or email without the user’s request.
Unrecognized Logins and Notifications
Instagram provides users with login activity details. If a user notices logins from unfamiliar devices or locations, it could indicate a breach. Users are urged to monitor their login activity regularly and report any discrepancies immediately.
Issues with Login Credentials
Difficulty logging in to an account can also signal hacking. If a user receives password reset requests that they did not initiate or is unable to access their account due to incorrect credentials, it’s essential to take immediate action.
Effective Recovery Steps After Hacking
Initial Actions to Secure Your Account
If a user’s Instagram account has been hacked, the first step is to secure any links to other social media accounts or email addresses associated with Instagram. Users should immediately change passwords for all accounts if they fear a breach.
How to Recover Access to Your Hacked Instagram Account
Recovering a hacked Instagram account involves specific steps:
- Use Instagram’s Recovery Options: Go to the login screen and click on “Forgot password?” Follow the on-screen instructions to receive a password reset email.
- Verify Your Identity: Instagram may require identification verification. Be prepared to provide the original email or phone number associated with the account.
- Secure Your Account: Once access is restored, immediately change the password and enable two-factor authentication to prevent future breaches.
Notifying Your Followers and Friends
After regaining access, it’s crucial to inform followers about the breach. This action can prevent hackers from misusing the account to favor their malicious activities. Users should also encourage followers to ignore any suspicious messages received from the account during the hacking period.
Preventive Measures Against Instagram Hackers
Best Security Practices for Instagram Users
Preventing hacking incidents is far better than dealing with the aftermath. Here are some best practices:
- Use Strong Passwords: Users should create unique passwords that combine letters, numbers, and symbols. Avoid using personal information that can be easily guessed.
- Limit Account Sharing: Avoid sharing account credentials, even with close friends or family, as this can lead to unintentional breaches.
- Monitor Account Activity: Regularly check login activity and ensure no unrecognized logins have occurred.
The Role of Two-Factor Authentication
Two-factor authentication (2FA) adds an additional security layer that requires not only a password but also a secondary method of verification, such as a code sent via SMS or email. Enabling 2FA greatly reduces the chances of unauthorized access and is highly recommended for all users.
Regularly Updating Passwords
Changing passwords periodically is a good practice that increases security. Consider updating passwords every few months and avoiding the use of the same password across multiple platforms.
Future of Instagram Security
Emerging Trends in Cybersecurity for Social Media
The landscape of cybersecurity is evolving rapidly. As social media platforms grow, hackers are becoming more sophisticated, utilizing advanced techniques for breaching security. Emerging trends include:
- Artificial Intelligence: AI tools are being developed to detect and prevent hacking attempts in real time.
- Biometric Verification: Beyond passwords, biometric methods such as fingerprint or facial recognition are gaining traction.
- Behavioral Analytics: Tools that analyze user behavior patterns offline and online can detect anomalies that indicate a potential hack.
Instagram’s Ongoing Efforts to Combat Hacking
Instagram continues to enhance its security measures. Regular updates and patches to the platform’s algorithms help thwart potential hacking attempts. Furthermore, Instagram provides users with resources dedicated to account security, helping them recognize threats and take proactive measures.
How Users Can Stay Informed
Staying informed about the latest trends in cybersecurity and Instagram updates is vital. Users should:
- Follow official Instagram blogs and support pages.
- Stay active in online communities discussing cybersecurity issues.
- Attend webinars and read articles from reputable sources to constantly update their knowledge on maintaining security.