Harnessing Agentic AI: Strategies for Enhanced Business Performance
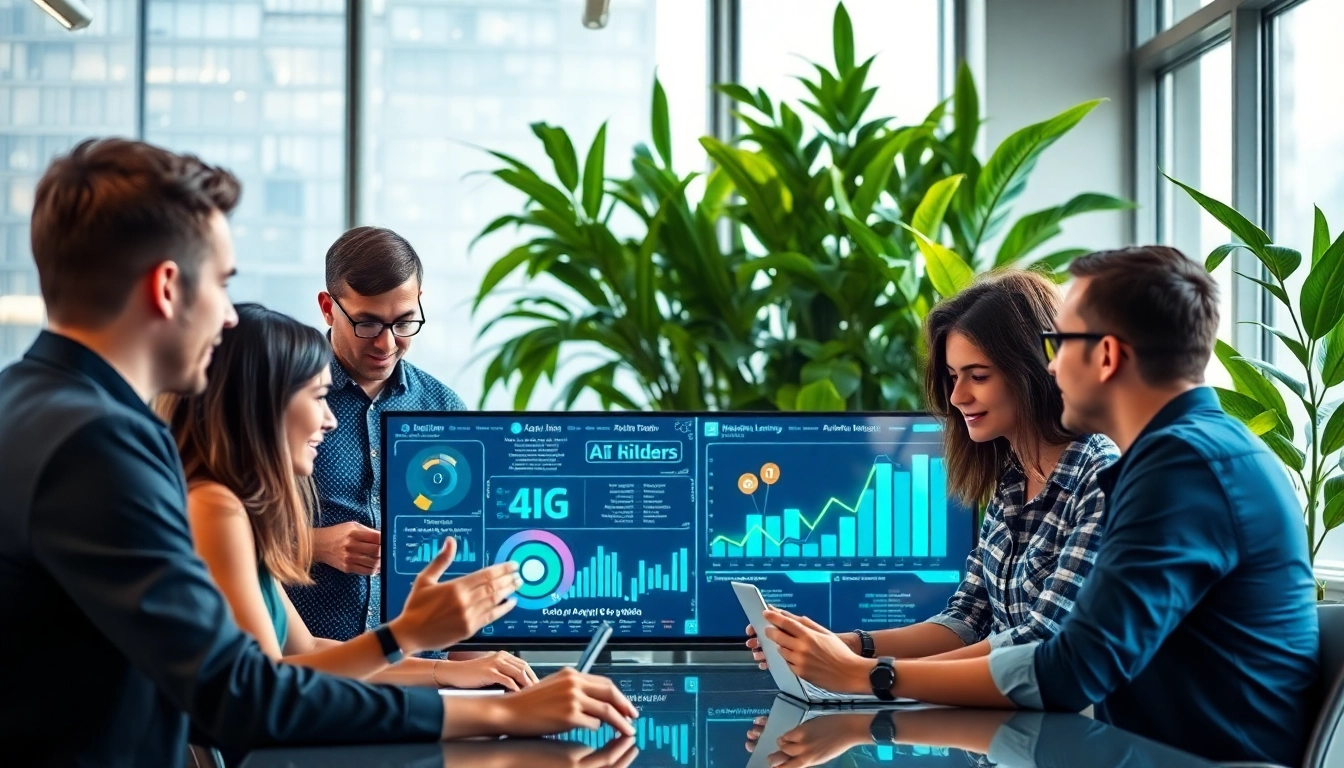
Understanding Agentic AI
Defining Agentic AI and Its Applications
Agentic AI, a term that has recently gained traction in the sphere of artificial intelligence, refers to systems designed to exhibit autonomous decision-making capabilities akin to that of human agents. Unlike traditional AI systems, which typically operate under predefined algorithms and limit their actions to a narrow set of parameters, agentic AI can generate contextual responses, learn from experiences, and navigate complex situations more effectively. This enhancing capacity means agentic ai holds notable potential across various sectors, from healthcare to finance and supply chain management.
In practical applications, agentic AI can perform tasks such as real-time data analysis, personalized content delivery, predictive maintenance, and customer interaction. Its autonomous nature empowers organizations to automate decisions that require understanding user preferences, market trends, or operational efficiencies. For instance, in healthcare, agentic AI can analyze patient histories to recommend treatment plans, while in finance, it can assess risk and detect fraud with far greater accuracy than traditional models.
How Agentic AI Differs from Traditional AI
While traditional AI is built upon data-driven algorithms and predefined rules, agentic AI enhances these capabilities by incorporating elements of autonomy and contextual awareness. Traditional systems often follow a fixed pathway, focusing on problem-solving based only on historical data. In contrast, agentic AI leverages machine learning techniques and reinforcement learning to adapt its decision-making process based on feedback and changing variables.
For example, consider a recommendation engine using traditional AI. It relies on past user behaviors to suggest products. However, if it encounters a new user profile without historical data, its effectiveness can diminish. Agentic AI, on the other hand, can observe patterns in real-time and adapt its recommendations, making it more effective in dynamic environments. This autonomy allows for continuous improvement, as the system ‘learns’ from its interactions and adjusts its actions accordingly.
Benefits of Implementing Agentic AI in Businesses
Implementing agentic AI offers myriad advantages for businesses looking to enhance their operational efficiency and customer engagement:
- Increased Efficiency: Automating complex tasks reduces the burden on human resources, allowing teams to focus on higher-level strategic planning.
- Improved Decision Making: With the ability to analyze vast amounts of data quickly, agentic AI can provide insights into market trends, customer behavior, and operational bottlenecks.
- Enhanced Customer Experience: Through personalized and timely responses, businesses can foster more meaningful customer relationships and higher satisfaction rates.
- Scalability: The adaptable nature of agentic AI solutions allows businesses to scale operations quickly, responding to growth opportunities without the proportional increase in costs.
- Innovation: Agentic AI encourages innovation by providing insights that can lead to the development of new products and services based on evolving consumer needs.
Challenges in Adopting Agentic AI
Identifying Common Implementation Obstacles
As businesses explore the integration of agentic AI technologies, they may encounter several challenges that can impede effective implementation:
- Complexity in Integration: Incorporating agentic AI into existing systems can require substantial changes to IT infrastructure, which may involve significant time and resources.
- Skill Gaps: Organizations may lack personnel with the requisite knowledge and skills to develop and maintain agentic AI systems, necessitating additional training or hiring.
- Resistance to Change: Employees may be reluctant to adopt new technologies, fearing job displacement or being overwhelmed by unfamiliar processes.
- Data Quality and Access: High-quality data is essential for training agentic AI; businesses must ensure their data is clean, accessible, and structured appropriately to yield the best results.
Addressing Data Privacy Concerns
With the capacity for agentic AI to analyze and learn from vast datasets, data privacy emerges as a paramount concern. Companies adopting this technology must prioritize establishing robust protocols to safeguard sensitive information. This can include implementing data anonymization techniques, ensuring compliance with regulations such as GDPR or HIPAA, and providing transparency about how data is collected and used.
Organizations should also consider adopting an ethical framework for AI usage, ensuring that agentic AI operates fairly, transparently, and accounts for biases in decision-making. By doing so, businesses can nurture trust among consumers and prevent potential legal implications resulting from mismanaged data privacy.
Managing Change and Employee Skepticism
Resistance to change within an organization is a common challenge when implementing new technologies. Employees may feel threatened by the introduction of agentic AI systems, leading to skepticism about their role and the future of their jobs. To manage this change effectively, businesses should prioritize open lines of communication throughout the integration process.
Engaging employees in the transition can help mitigate fears. This includes providing thorough training programs that demonstrate the benefits of agentic AI, showcasing how it complements rather than replaces their contributions. By involving employees in the dialogue and addressing their concerns directly, organizations can cultivate a culture of acceptance and collaboration.
Best Practices for Effective Agentic AI Integration
Steps for Seamless Deployment
To ensure a successful deployment of agentic AI, organizations should follow these best practices:
- Define Clear Objectives: Clearly articulated goals for the AI implementation project guide development and assess its impact on business processes.
- Choose the Right Technology: Selecting the appropriate tools and platforms is crucial, as not all agentic AI solutions will align with an organization’s unique requirements.
- Establish a Pilot Program: Start with a smaller, controlled implementation to assess feasibility and effectiveness before scaling the project.
- Monitor and Adapt: Continuous monitoring allows for adjustments based on real-time feedback, ensuring the AI system evolves effectively over time.
Training Teams to Leverage Agentic AI
Training employees to work with agentic AI technologies is vital to unlocking their full potential. Organizations should invest in comprehensive training programs that demystify AI concepts and emphasize the practical applications relevant to different departments.
Hands-on workshops can facilitate better understanding and offer opportunities for employees to practice using AI tools in real-world scenarios. Additionally, creating a knowledge-sharing platform where employees can discuss challenges, discoveries, and best practices can further enhance team competency and foster innovation.
Measuring Impact and Success Metrics
Establishing metrics to measure the success of agentic AI implementation is essential for justifying investments and optimizing deployment strategies. Key performance indicators (KPIs) might include:
- Efficiency Gains: Measure reductions in time or costs as a result of automation and improved processes.
- Customer Satisfaction Rates: Analyze feedback and engagement metrics to assess improvements in user interactions.
- Revenue Impact: Evaluate how agentic AI has influenced sales through better customer targeting and personalization.
- Employee Productivity Levels: Monitor any shifts in employee productivity resulting from reduced manual workloads or enhanced decision-making capabilities.
Real-World Success Stories of Agentic AI
Case Studies of Companies Implementing Agentic AI
Numerous organizations have successfully implemented agentic AI, driving significant impact on their operations. One notable case is that of a global retail chain that incorporated agentic AI into its supply chain management system. By utilizing predictive analytics, the company was able to reduce inventory levels by 30% while ensuring a consistent product availability. Through enhanced data insights, the company effectively optimized its logistics processes, illustrating the potential efficiencies agentic AI can realize.
Another example involves a leading hospital that deployed agentic AI to manage patient triage. By using AI-driven algorithms to analyze symptoms, historical data, and patient inflow, the hospital improved its response times by 25%, increasing overall patient satisfaction and outcomes. Such case studies underscore the transformative benefits of adopting agentic AI in various industry contexts.
Lessons Learned from Successful Integrations
Successful integrations of agentic AI yield valuable lessons applicable to other organizations:
- Pilot Before Full Scale: Testing technologies on a small scale before widespread implementation can provide crucial insights and mitigate risks.
- Continuous Training is Essential: Ongoing employee education is paramount to maintain an agility that aligns with evolving AI capabilities and market dynamics.
- User-Centric Design Matters: Solutions built with user experience in mind yield better engagement and adoption rates, helping to overcome resistance and skepticism.
Key Takeaways for Future Implementations
For organizations looking to adopt agentic AI, it is critical to remember the importance of a clear strategy, stakeholder engagement, and data integrity. Alignment with business goals, alongside consideration for ethical implications and employee concerns, will aid in fostering a successful-agentic AI integration journey. Moreover, being adaptable and responsive to new information ahead of market trends ensures continued relevance in an ever-evolving technological landscape.
The Future of Agentic AI in Business
Emerging Trends and Innovations
The landscape of agentic AI is rapidly evolving as technological advancements introduce innovative capabilities. One trend gaining momentum is the integration of natural language processing (NLP) within agentic AI systems, allowing for more human-like interactions between machines and users. This capability will enhance customer service applications, enabling AI systems to understand and respond to inquiries more naturally.
Furthermore, as businesses prioritize sustainability, agentic AI is expected to play a crucial role in optimizing resource consumption patterns and supporting corporate social responsibility initiatives through more efficient operational models.
Predictions for Market Impact
Experts predict that the demand for agentic AI technologies will continue to surge, catalyzing significant shifts across multiple industries. The increased reliance on data-driven decision-making is likely to create a competitive landscape where businesses adopting agentic AI first will gain substantial market advantages. Furthermore, advancements in machine learning algorithms are expected to enhance the predictive and prescriptive capabilities of agentic systems, driving further adoption.
Preparing for a More Agentic AI-Driven Landscape
Looking ahead, organizations must prepare for a landscape increasingly dominated by agentic AI. This includes not only investing in technology but also fostering a culture that embraces innovation and change. Organizations should regularly evaluate their AI strategies in light of emerging trends while aligning initiatives with broader business objectives. Lastly, proactive engagement with stakeholders at all levels of the organization will provide the necessary frameworks to navigate the complexities of agentic AI evolution effectively.
Leave a Reply